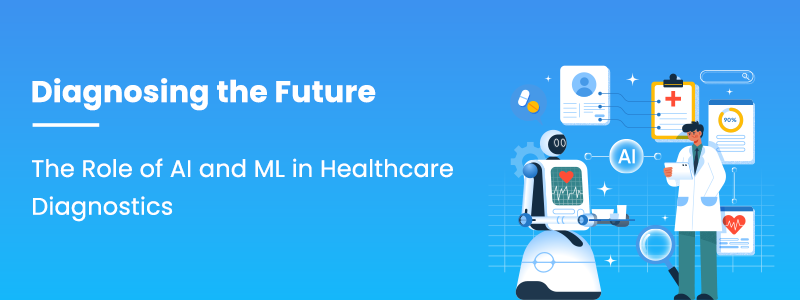
Artificial intelligence isn’t one technology, however alternatively a set of them. Most of these technologies have instantaneous relevance to the healthcare discipline, however, the appropriate procedures and tasks they help vary widely.
The destiny of healthcare diagnostics is deeply intertwined with the improvements in artificial intelligence (AI) and system getting to know (ML). These technologies are revolutionizing how medical situations are detected, diagnosed, and handled. Here are a few key components in their function in healthcare diagnostics:
1. Early Detection and Diagnosis: &;AI and ML algorithms can examine great portions of clinical statistics, together with affected person facts, imaging scans, genetic records, and even actual-time physiological information from wearable gadgets. This functionality allows earlier detection and greater accurate analysis of illnesses including most cancers, heart conditions, and neurological problems.
2. Personalized medicine: AI and ML can learn about said individuals and tailor decisions based entirely on genetics, lifestyle factors, and a number of customized variables. This approach, called precision medicine, improves the effectiveness of treatment and reduces adverse effects by targeting interventions at the appropriate needs of each individual patient on the snow.
3. Medical image analysis: AI algorithms are capable of interpreting clinical images of X-rays, MRIs, CT scans, etc. with accuracy that matches or possibly exceeds that of human experts This enables the diagnosis of conditions ranging from tumors to tumors rapidly and specifically, improving outcomes for affected individuals, . and reduced health care costs.
4. Predictive Analytics: By analyzing historical patient data, AI and ML can identify patterns and trends that help predict future health risks and outcomes. This enables proactive interventions to prevent or manage chronic conditions, leading to better long-term health outcomes and reduced healthcare spending.
For any further information / query regarding Technology, please email us at info@varianceinfotech.in
OR call us on +1 630 534 0223 / +91-7016851729, Alternately you can request for information by filling up Contact Us
5. Drug Discovery and Development:AI and ML algorithms are increasingly used in drug discovery and development processes. They can analyze vast molecular datasets to identify potential drug candidates, predict their efficacy and safety profiles, and optimize treatment regimens for individual patients.
6. Remote Monitoring and Telemedicine: AI-powered monitoring systems can continuously track patients’ health metrics remotely, alerting healthcare providers to potential issues before they escalate. Combined with telemedicine platforms, this enables more efficient and accessible healthcare delivery, especially in rural or underserved areas.
7. Clinical Decision Support: AI and ML algorithms can provide clinicians with real-time decision support by analyzing patient data and recommending diagnostic tests, treatment options, and care plans. This helps healthcare providers make more informed decisions, reducing diagnostic errors and improving patient outcomes.
8. Ethical and Regulatory Considerations: As AI and ML technologies become more integrated into healthcare diagnostics, it’s crucial to address ethical concerns such as patient privacy, algorithm bias, and transparency in decision-making. Robust regulatory frameworks are needed to ensure the responsible and equitable use of these technologies while safeguarding patient rights and well-being.
Machine learning – neural networks and deep learning:
Machine learning is a statistical technique for fitting models to data and to ‘learn’ by training models with data. Machine learning is one of the most common forms of AI; in a 2018 Deloitte survey of 1,100 US managers whose organisations were already pursuing AI, 63% of companies surveyed were employing machine learning in their businesses.1 It is a broad technique at the core of many approaches to AI and there are many versions of it.
In healthcare, the most common application of traditional machine learning is precision medicine – predicting what treatment protocols are likely to succeed on a patient based on various patient attributes and the treatment context.2 The great majority of machine learning and precision medicine applications require a training dataset for which the outcome variable (eg onset of disease) is known; this is called supervised learning.
A more complex form of machine learning is the neural network – a technology that has been available since the 1960s has been well established in healthcare research for several decades3 and has been used for categorisation applications like determining whether a patient will acquire a particular disease. It views problems in terms of inputs, outputs and weights of variables or ‘features’ that associate inputs with outputs. It has been likened to the way that neurons process signals, but the analogy to the brain’s function is relatively weak.
The most complex forms of machine learning involve deep learning, or neural network models with many levels of features or variables that predict outcomes. There may be thousands of hidden features in such models, which are uncovered by the faster processing of today’s graphics processing units and cloud architectures. A common application of deep learning in healthcare is recognition of potentially cancerous lesions in radiology images.4 Deep learning is increasingly being applied to radiomics, or the detection of clinically relevant features in imaging data beyond what can be perceived by the human eye.5 Both radiomics and deep learning are most commonly found in oncology-oriented image analysis. Their combination appears to promise greater accuracy in diagnosis than the previous generation of automated tools for image analysis, known as computer-aided detection or CAD.
Deep mastering is likewise an increasing number of used for speech popularity and, as such, is a form of herbal language processing (NLP), described beneath. Unlike earlier types of statistical analysis, every characteristic in a deep getting to know model normally has little meaning to a human observer. As a end result, the reason of the version’s effects can be very hard or not possible to interpret.
Conclusion:
Overall, AI and ML hold tremendous promise for increasing the speed, accuracy, and efficiency of healthcare research, ultimately leading to more personalized care and impactful outcomes. Understanding this potential, however, requires collaboration among stakeholders, including healthcare vendors, researchers, policymakers and once developers, to overcome challenging situations and the benefits of this revolutionary technology have been fully exploited.
Do You Need more information?
For any further information / query regarding Technology, please email us at info@varianceinfotech.in
OR call us on +1 630 534 0223 / +91-7016851729, Alternately you can request for information by filling up Contact Us
Leave a Reply